In an era where data is hailed as the new oil, the ability to harness this invaluable resource effectively is what sets successful enterprises apart. Picture a bustling hub of activity, akin to a thriving city. Now, visualize data as the lifeblood coursing through the veins of this city, connecting every nook and cranny. This is the essence of data integration – it’s about orchestrating a symphony from the cacophony of data emanating from myriad sources.
According to a noteworthy statistic, organizations employing data integration witness a striking 23% uptick in revenue. This isn’t a mere coincidence but a testament to the transformative power of having a unified view of your data. It’s about elevating decision-making, bolstering business intelligence, and driving revenue growth.
Embark on a journey through the realms of data integration with this comprehensive guide. Whether you’re keen on understanding the ETL (Extract, Transform, Load) process, exploring data integration platforms, or delving into the use cases across various sectors, we’ve got it all covered. Ready to unlock the treasure trove of data integration? Let’s dive in.
What is data integration?
Data integration is a pivotal process that amalgamates data from diverse source systems into a unified view, enabling a comprehensive analysis. By leveraging potent data integration tools, organizations pave the way for robust business intelligence initiatives, which in turn, steer informed decisions and streamline business operations.
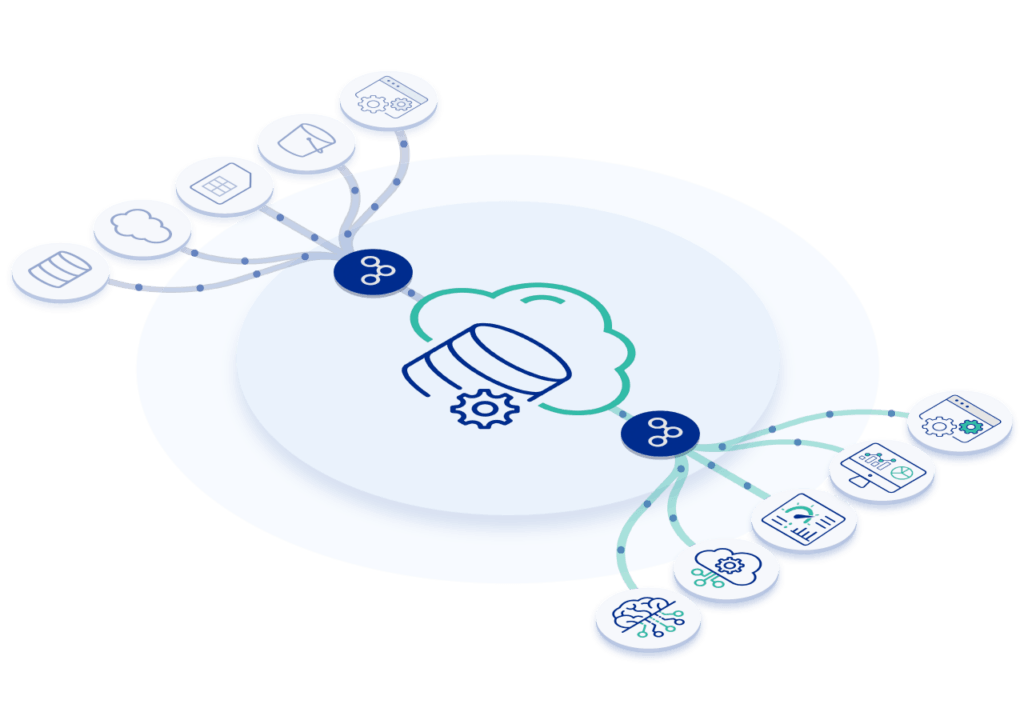
Why is data integration imperative?
In the modern data-centric landscape, data integration is the knight in shining armor, ensuring a ceaseless flow of data across different systems within an enterprise. It’s akin to having a super-efficient traffic system in a bustling city, where data flows smoothly without bottlenecks, making crucial information readily available for analysis. This boosts an organization’s decision-making prowess, showcasing the undeniable importance of a well-orchestrated data integration process in today’s enterprises.
What are some data integration techniques?
Data integration is a pivotal process in any organization’s IT strategy, involving various techniques each suited to specific needs and outcomes. Understanding these techniques is crucial for effective data management and decision-making.
Data Consolidation
Data consolidation is the process of combining data from multiple sources into a single, centralized repository, typically a data warehouse.
- Benefit: This method simplifies data analysis and reporting by providing a comprehensive view of combined data.
- Use Case: A retail company consolidating sales data from different regional stores to analyze overall performance.
Data Propagation
This involves replicating data from one location to another using tools such as ETL (Extract, Transform, Load).
- Advantage: It is essential for maintaining up-to-date and synchronized data across different systems.
- Use Case: A financial institution using ETL to synchronize customer data between its CRM system and transaction databases.
Data Virtualization
Data virtualization creates an abstract, integrated view of data from various sources, without the need for physical consolidation.
- Strength: Offers agility and real-time data access, ideal for dynamic business environments.
- Use Case: A healthcare provider offering doctors real-time access to patient records from various databases for better diagnosis and treatment plans.
Each of these techniques has a specific role in the broader data integration landscape, providing businesses with the flexibility to choose the most appropriate method based on their unique data architecture and integration requirements.
As we have explored, data integration encompasses a variety of techniques, each tailored to specific organizational needs. Data consolidation, propagation, and virtualization are just the tip of the iceberg. Moving beyond these foundational methods, let’s delve into the realm of data federation, another pivotal aspect of data integration that offers unique advantages in managing and accessing disparate data sources.
What is Data Federation vs Data Integration?
Data Federation is an integral technique in data integration, enabling a unified view of diverse, distributed data sources. Unlike methods that physically consolidate data, data federation creates a virtual database, allowing users to access and retrieve data as if from a single entity. This approach simplifies data analysis and management, particularly for large organizations dealing with data spread across multiple systems. It stands as a critical component in the broader field of data integration, offering unique advantages in terms of flexibility and efficiency in handling complex datasets.
In “Data Federation,” the term “federation” refers to the idea of bringing together data from different sources and making it accessible as if it were all from one place, without actually moving or copying the data. It’s like having a single library catalog that shows books from many libraries, even though the books remain in their original locations.
Understanding data federation enhances our comprehension of the diverse strategies available in data integration. With this knowledge, we can better navigate the myriad of tools employed in this field. Next, let’s transition to examining these tools, the skilled navigators that effectively steer our data from source to destination, ensuring seamless integration and accessibility.
What tools are employed in data integration?
Embarking on the voyage of data integration involves navigating through a sea of tools and platforms. These data integration platforms, like SnapLogic, are the skilled captains steering the ship amidst a storm of data, ensuring a safe passage from source to destination. With features to automate the ETL process, they offer connectors to a wide array of data sources like SQL databases, CRMs, and more, bridging the chasms between disparate data silos.
Modern data integration tools have evolved to embrace new challenges. They now cover ELT operations, real-time data integration, data virtualization, and more, catering to the burgeoning demands for faster, more flexible, and scalable data management solutions.
How does data integration enhance business intelligence?
At the core of data integration lies a treasure trove of enriched business intelligence (BI). It’s like having a crystal ball that provides a panoramic view of operations, customer behaviors, and market trends. The insights unearthed are the compass guiding organizations toward informed business strategies and competitive prowess.
Moreover, data integration extends its utility across various use cases in different business sectors. Whether it’s optimizing supply chains, enhancing customer experiences, or fostering innovation, the narrative of success is often written with the ink of well-executed data integration.
How is API Management integral to data integration?
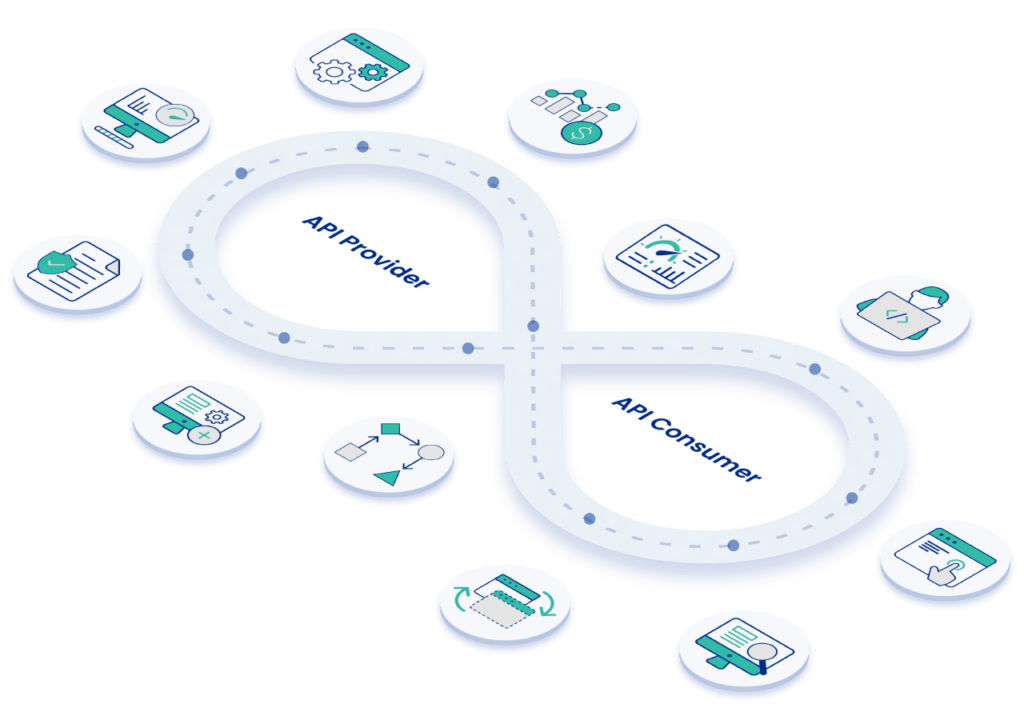
API Management is a facet of data integration that cannot be overlooked. Efficient API management ensures seamless communication between different applications and data sources, thus forming a crucial cog in the data integration machinery. Platforms like SnapLogic provide robust API Management solutions to create, manage, and secure all your APIs at scale, thus bolstering the data integration infrastructure.
The mechanics of data integration
Navigating the realm of data integration is like piecing together a complex puzzle where each piece is a fragment of data. When correctly assembled, a clear picture emerges, revealing insights that propel businesses forward. For instance, a report by Talend states that companies with advanced data integration capabilities are 3 times more likely to be extremely competitive.
What are ETL and ELT processes?
The cornerstone of data integration lies in the processes of ETL (Extract, Transform, Load) and ELT (Extract, Load, Transform). ETL is about extracting data from varied source systems, transforming it to a standardized format, and then loading it into a target system, say, a data warehouse. On the flip side, ELT is a more modern approach where data is extracted, loaded into the target system, and then transformed for analysis. For example, ELT can be particularly beneficial in cloud-native environments due to its ability to leverage the scalable compute resources of the cloud.
How do data sources, source systems, and target systems interact?
The saga of data integration begins with data sources and source systems which could range from databases, ERP systems to flat files. The target system is where the consolidated data finds its home, ready to be analyzed. For instance, integrating data from Salesforce (a source system) with a central data warehouse (target system) to achieve a 360-degree view of customer interactions.
How is data management addressed?
The realm of data management is vast, encompassing data quality, data governance, and master data management. For example, a data governance initiative could help in achieving regulatory compliance, while master data management ensures consistency of data across the organization.
What is the essence of data warehousing and data lakes?
The havens for integrated data are data warehouses and data lakes. While data warehouses are structured and tailored for analytical processing, data lakes are vast reservoirs storing data in its raw form. For instance, a data lake might store data from IoT devices, ready to be analyzed for patterns.
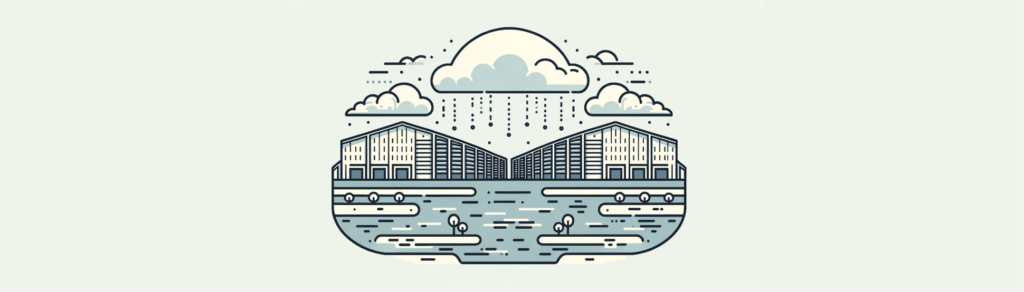
How do data pipelines, data transformation, and data virtualization contribute?
Imagine data pipelines as the highways facilitating the journey of data from source to target. Data transformation is akin to translating languages, ensuring data from diverse sources speaks a common dialect. Data virtualization, on the other hand, is like having a universal library card to access data scattered across various libraries without moving them.
What role do data engineers, connectors, and middleware play?
Data engineers are the architects, connectors are the bridges, and middleware is the translator in the realm of data integration. They ensure that data flows seamlessly, is correctly transformed, and can be accessed and exchanged across different systems.
How is a unified view achieved?
A unified view is the Holy Grail in data integration, achieved by harmonizing data from diverse systems and sources. It’s like having a clear lens to view a multifaceted diamond, each facet representing a different data source.
Advanced topics and emerging trends in data integration
As the digital realm evolves, so does the sphere of data integration. Let’s explore the avant-garde topics and burgeoning trends shaping the modern data integration landscape.
Real-time data integration: streaming and on-demand
Real-time data integration, facilitated by streaming data technologies, allows for on-demand access to data. This real-time access is instrumental in making informed decisions swiftly, a critical advantage in today’s fast-paced business environment.
Cloud-based integration: SaaS and on-premises solutions
The cloud has revolutionized data integration. Cloud-based solutions, including SaaS platforms, offer scalable, cost-effective alternatives to traditional on-premises solutions, providing the flexibility and accessibility modern enterprises crave.
Big data integration: unstructured data and scalability
Big data integration addresses the challenges posed by the sheer volume and variety of data, including unstructured data. Scalability is paramount to handle the exploding data volumes while ensuring performance.
IoT, API, and application integration
The interplay between IoT, API, and application integration is weaving a new narrative in data integration. These technologies are fostering seamless connectivity and data exchange, driving innovation and efficiency.
Machine learning, analytics, and data-driven processes
Machine learning and analytics tools are the linchpins in deriving actionable insights from integrated data. These technologies, coupled with data-driven business processes, are the driving force behind modern, intelligent enterprises.
Notable providers and open source options
The market is brimming with providers offering robust data integration tools and solutions. From notable vendors to open-source options, organizations have a wide array of choices to meet their unique data integration needs.
What are the different types of data integration?
Data integration can be divided into different types based on how and why data is being moved, stored, and used.
1. Data migration
Data migration is the process of moving data assets from one platform to another, making it a form of integration. You’ll hear this term when data is being moved from an on-premises database to a cloud-based one or vice versa. Migration also applies to moving data assets from one application to another.
2. Master data management (MDM)
Master data management (MDM) is the process of creating a single dataset for the whole organization to use. The dataset serves as a single source of truth for your organization and is called master data. It contains information on the core entities of a business, such as the customers, products, services, location, and pricing. For MDM, a robust integration model is needed. That’s because data from internal and external sources has to be reconciled, enriched, and de-duplicated before it goes into the master dataset.
3. Enterprise application integration (EAI)
Just like the name suggests, EAI refers to the integration between different applications and databases. You need data from within your tech stack to flow from one app to another, such as customer data going from HubSpot to Salesforce or employee data going from your LMS to your HRIS. To achieve that, you integrate those apps together using built-in, custom, or third-party integrations. All such integrations fall under the category of EAI. EAI is critical because it helps create omnichannel customer experiences.
4. Data aggregation
Data aggregation is the process of gathering and compiling data to be either stored in its raw form or prepared for analytics. Think of a marketing campaign that uses email, social media, and pay-per-click advertising to promote an online event. The data about each marketing channel will live originally in separate tools, so it will need to be aggregated into a single report or dashboard before the overall performance of the campaign can be analyzed.
5. Data federation
Data federation is the process of creating a virtual database that shows an integrated view of data. The virtual database does not store the data, only information on the data’s location.
6. Data lake
A data lake stores massive amounts of raw data that has not been given a purpose or prepared for usage. It is a result of integration between multiple external and internal sources of data. This data may or may not eventually be used, but it is stored and held for its potential value. It is a repository for unstructured and structured data alike.
7. Data warehousing
Data warehouses store structured data from multiple sources. This data first goes through the ETL process. It is loaded into the data warehouse, where it is then used for reporting, analytics, business intelligence, and data virtualization. Examples of popular data warehouses include AWS Redshift, Microsoft Azure SQL Data Warehouse, Snowflake, and SAP Datasphere.
Software integration vs data integration
Software integration and data integration are essential processes in the IT landscape, catering to different requirements and objectives.
Software Integration: Software integration involves connecting various software systems to enable them to work together as a cohesive unit. This process is crucial for creating a unified workflow where data and processes can flow seamlessly across different software applications. Examples include integrating a Customer Relationship Management (CRM) system with an Enterprise Resource Planning (ERP) system to ensure smooth information exchange and process alignment.
Data Integration: On the other hand, data integration pertains to the process of gathering, cleansing, and consolidating data from various sources to provide a unified view. This is crucial for making informed decisions as it ensures that data is accurate, consistent, and accessible. Data integration often involves processes like Extract, Transform, Load (ETL) or Extract, Load, Transform (ELT), data warehousing, and data lake formations.
Comparison:
- Objective:
- Software integration aims at enabling interoperability among different software systems to streamline business processes.
- Data integration aims at amalgamating data from different sources to provide a unified, accurate, and accessible view of the data.
- Process:
- Software integration may require creating new APIs, middleware, or utilizing existing connectors to ensure smooth communication between systems.
- Data integration usually involves ETL/ELT processes, data transformation, and data quality assurance to ensure the data is ready for analysis.
- Outcome:
- Through software integration, organizations can achieve improved workflow efficiency, reduced data redundancy, and better customer experiences.
- Through data integration, organizations are better positioned to perform analytics, generate insights, and support decision-making.
Both processes, while distinct, can complement each other in an organization’s endeavor to achieve a streamlined operational environment and data-driven decision-making.
What are the different methods of integrating data?
So, how do you integrate your data? There are several approaches, ranging from manual integration to data integration platforms. Sometimes, these methods are used in combination to build customized integration architectures.
Manual integration
Manual integration can be achieved by using point-to-point integration. In this model, developers integrate different apps and databases using custom code and application programming interfaces (APIs).
Uniform access
In uniform access integration, developers set up customized dashboards and reports that pull data from multiple sources and present them to the data analyst in a unified view. This can be done through custom software or SaaS solutions. The original data stays at its source and is only replicated at the time of the analysis.
This type of integration does not require a lot of storage and is highly scalable. But analysts do need to make data access requests with this type of integration, which puts a lot of strain on the servers.
Common storage
Common storage data integration uses the same methods as uniform access integration, with the addition of a data warehouse. Data from multiple sources is pulled in, transformed, and stored in a warehouse. The data can then be used for analysis.
You don’t need to make multiple data access requests with common storage, reducing the load on your servers. But you need to invest in on-premises or cloud-based data storage.
Middleware integration
Any software system that serves as a bridge for data to travel from one app or database to another is called middleware. With the right middleware, you can integrate every application in your tech stack.
iPaaS
Integration through integration platform as a service (iPaaS) is a type of middleware integration. iPaaS comes with advanced capabilities to serve as an integration hub for all of your organizational data. Other types of middleware may only serve as a bridge between two apps. Integration platforms like SnapLogic help you create workflows, uphold data quality standards, and take complete control of your integration architecture.
What should you look for in a data integration solution?
Selecting a data integration solution is a pivotal decision. The right choice can accelerate your data processes, ensuring accuracy and ease with the aid of Artificial Intelligence (AI) and Machine Learning (ML).
Here are crucial considerations to ponder upon:
- Scalability: As your data empire expands or new third-party apps and databases join the fray, can the solution scale up or out seamlessly? A statistic by IDC projected that the collective sum of the world’s data will grow to 175 zettabytes by 2025, underlining the need for scalable solutions.
- Environment Management: Can the platform adeptly manage both public and private clouds along with on-premise environments? A hybrid integration could cater to integrating datasets from a myriad of environments.
- Ease of Integration: Does it champion a no-code philosophy, enabling click-not-code integrations? Such an approach simplifies building and editing workflows, making the process less daunting for your team.
- User-Friendly yet Robust: While being robust for developers, is it user-friendly for your business teams? A balanced solution facilitates both technical and non-technical personnel in managing your integration architecture.
- Industry Recognition: Has the solution received accolades or favorable reviews from reputable industry analysts? Such recognition can be a testimony to its competency and reliability.
Mastering data integration: from ingestion to replication
Data integration is a journey, commencing from the point you get data from different sources to when it’s ready for analytics. It’s about harmonizing enterprise data, embracing methods like change data capture to track data changes. Your data integration system should adeptly handle data modeling to define data relationships, and data replication to duplicate data across systems.
A robust data store is imperative, where data is ready to be read and analyzed. The process of data ingestion is crucial, marking the entry of data into the system. Various data integration methods and techniques are employed to ensure smooth data transition, be it into a relational database or other platforms, refining the data for insightful analytics.
What are integration patterns?
Integration patterns are standardized solutions to common integration challenges. They provide a blueprint for solving particular problems in a robust and maintainable manner. Some prevalent patterns include Batch Data Synchronization, Migration, Broadcast, Correlation, Aggregation, and Bi-directional Sync. Each pattern addresses specific scenarios, ensuring seamless data flow and integration across systems. For a deeper dive into integration patterns, their types, and real-world use cases, check out this comprehensive guide on Data Integration Patterns.
Generative integration and beyond
In the evolving landscape of data and software integration, the emergence of Generative Integration heralds a new era. By harnessing the power of Generative AI and Large Language Models, it not only streamlines the integration processes but also opens up possibilities for more intelligent, autonomous systems. As organizations stride towards a data-centric future, embracing such advanced integration methods will be pivotal. The blend of Software, Data, and Generative Integration will likely drive more cohesive, insight-driven enterprises, propelling them towards unprecedented levels of operational efficiency and informed decision-making, ready to tackle the complex challenges of tomorrow.
As Generative Integration evolves, tools like SnapGPT will likely be at the forefront, facilitating seamless connections between diverse systems and data realms. The road ahead is vibrant with the promise of intelligent integrations, orchestrating a symphony of data and applications that drive enterprise agility and innovation, heralding a new epoch of data-centric operational excellence.
Strengthen and simplify your data integrations with SnapLogic
The SnapLogic integration platform is here to make your data integration process more efficient and easier as a no-code solution. It offers hundreds of pre-configured integrations, and you can use drag-and-drop options to build more. AutoSuggest — an AI-powered integration assistant — also provides proven recommendations and guidance for smarter integration.